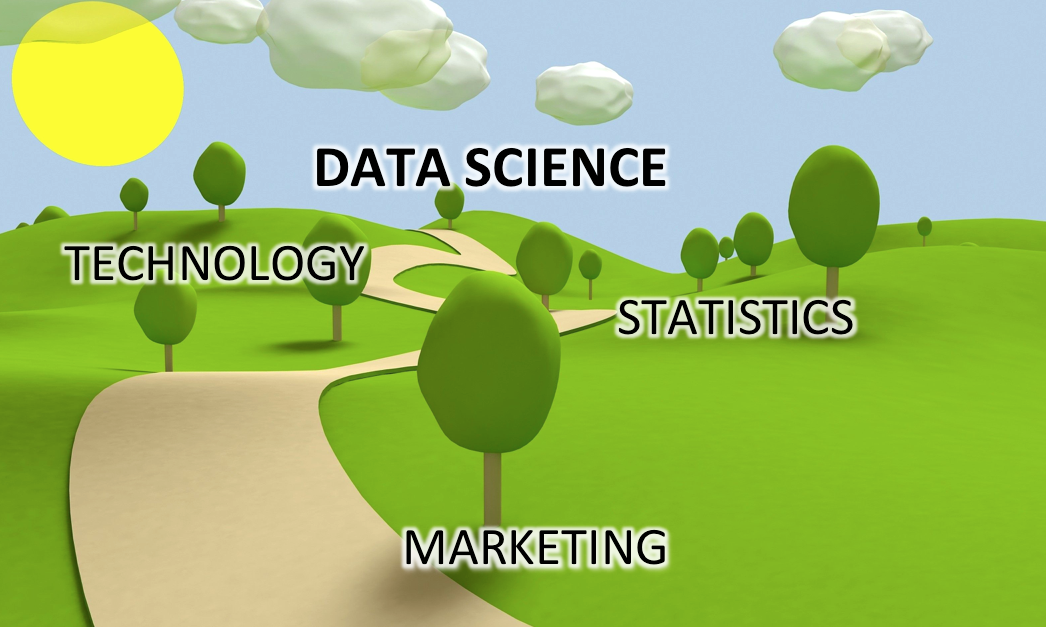
3 pathways to Data Science, and their strengths & weaknesses
As 4SIGHT SEARCH has helped build data analytics practices for organizations and traded industry stories, we’ve seen a few patterns. That said, our clients are typically in the Fortune 200 – 1000 space and/or marketing agencies . . . not Facebook, or Google. They have not been spoon-fed the best of Silicon Valley; they’ve had to bootstrap their analytics growth. Here’s a breakdown of what we see when internal practices stem from marketing, statisticians and/or IT groups.
#1. ANALYTICS STEMMED FROM MARKETING
This has been our most common scenario, given 4SIGHT’s marketing specialization. The analytics department has grown from marketing, first as digital campaign analytics, then into product analytics, and finally stepping into data science. Usually this occurs 1-2 hires at a time, and as the organization learns and service delivery is refined, data science evolves.
Here, the group’s strengths lie in translating the analytics into revenue, cross-functional agility, service delivery and ability to present data in captivating and resonating manners.
The common weaknesses lie in deeper scientific and statistical horsepower (reallyunderstanding the analytics), working with it efficiently, and overall technical ability (in thought and leveraging technology).
#2. ANALYTICS STEMMED FROM STATISTICIANS
In this instance, we’ve seen data experts build the internal practice, typically starting with a roll-up-your-sleeves leader who hires a 1-2 person staff.
Here, the group’s strengths lie in technical data knowledge, immediate understanding of how to “do data” (high volume, messy, raw data), and working with it quickly and comfortably.
The common weaknesses include lighter capabilities in optimization of the analytics, and digitalizing it. And not to generalize . . . but sometimes, they don’t always like to poke their heads above water, captivate a room and drive the business.
#3. ANALYTICS STEMMED FROM IT
Here, we have software engineers and DevOps maximizing the technology, living in Python, Java and R, and enabling their software to predict outcomes. This carries the group to a certain point. However, they quickly find themselves needing more marketing and revenue know-how, and they typically hire a dedicated statistician or two with strong business experience and academic training in mathematics or statistics.
No matter how you spin it and from where you grow it, these groups – like most – will have their sweet spots and thinner layers until the practice is fully-formed. This has been 4SIGHT’s experience and observation. How has your organization grown its practice? What has your org’s journey into data science (whatever that is, right?) been like, and where are you seeing your strengths and weaknesses?
EMPLOYERS: Sign up for our monthly employer newsletter & talent view here.
MARKETERS: Sign up for our monthly talent newsletter here.